What are the properties of maximum likelihood estimation?
Table of Contents
What are the properties of maximum likelihood estimation?
Maximum Likelihood Estimation (MLE) is a widely used statistical estimation method. In this lecture, we will study its properties: efficiency, consistency and asymptotic normality. MLE is a method for estimating parameters of a statistical model.
What are regularity conditions for MLE?
The regularity conditions include the following: the true parameter value θ must be interior to the parameter space, the log-likelihood function must be thrice differentiable, and the third derivatives must be bounded.
What is the meaning of MLE?

In statistics, maximum likelihood estimation (MLE) is a method of estimating the parameters of an assumed probability distribution, given some observed data. This is achieved by maximizing a likelihood function so that, under the assumed statistical model, the observed data is most probable.
What is the connection between a sufficient statistic and an MLE?
A theorem relating the two concepts indicates that if a maximum likelihood estimate (MLE) for a parameter is unique, then it is a function of every sufficient statistic.
What is MLE used for?
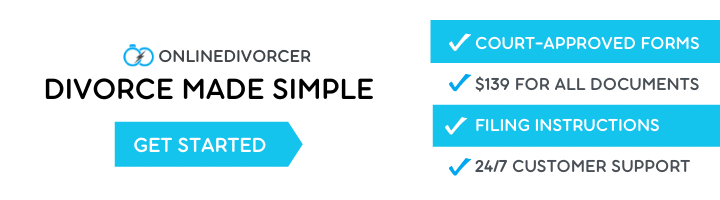
Maximum likelihood estimation involves defining a likelihood function for calculating the conditional probability of observing the data sample given a probability distribution and distribution parameters. This approach can be used to search a space of possible distributions and parameters.
What is MLE econometrics?
In statistics, maximum likelihood estimation (MLE) is a method of estimating the parameters of a statistical model given observations, by finding the parameter values that maximize the likelihood of making the observations given the parameters.
Where is MLE used?
We can use MLE in order to get more robust parameter estimates. Thus, MLE can be defined as a method for estimating population parameters (such as the mean and variance for Normal, rate (lambda) for Poisson, etc.) from sample data such that the probability (likelihood) of obtaining the observed data is maximized.
What is meant by invariance?
Definition of invariant : constant, unchanging specifically : unchanged by specified mathematical or physical operations or transformations invariant factor.
How do you find the variance of a maximum likelihood estimator?
This property is called asymptotic efficiency. I(θ) = −E [ ∂2 ∂θ2 ln L(θ|X) ] . Thus, the estimate of the variance given data x ˆσ2 = −1 / ∂2 ∂θ2 ln L(ˆθ|x). the negative reciprocal of the second derivative, also known as the curvature, of the log-likelihood function evaluated at the MLE.
Is the MLE a sufficient estimator?
If the MLE is itself sufficient, it is minimal sufficient. Rao–Blackwellization is never needed for the MLE. A statistic A = a(X) is ancillary if the distribution of A does not depend on θ. Intuitively, A then carries no information about θ.
What is maximum likelihood phylogenetic tree?
Maximum Likelihood is a method for the inference of phylogeny. It evaluates a hypothesis about evolutionary history in terms of the probability that the proposed model and the hypothesized history would give rise to the observed data set.
What is the purpose of MLE?
It involves maximizing a likelihood function in order to find the probability distribution and parameters that best explain the observed data. It provides a framework for predictive modeling in machine learning where finding model parameters can be framed as an optimization problem.
What is maximum likelihood estimation with example?
In the second one, θ is a continuous-valued parameter, such as the ones in Example 8.8. In both cases, the maximum likelihood estimate of θ is the value that maximizes the likelihood function. Figure 8.1 – The maximum likelihood estimate for θ….Solution.
θ | PX1X2X3X4(1,0,1,1;θ) |
---|---|
3 | 0 |