What is the direction of steepest ascent?
Table of Contents
What is the direction of steepest ascent?
In other words, the gradient ∇f(a) points in the direction of the greatest increase of f, that is, the direction of steepest ascent. Of course, the oppo- site direction, −∇f(a), is the direction of steepest descent. √ 16 − 4×2 − y2. The curve of steepest descent will be in the opposite direction, −∇f.
What is the difference between gradient descent and steepest descent?
Summary. The gradient is the directional derivative of a function. The directional of steepest descent (or ascent) is the direction amongst all nearby directions that lowers or raises the value of f the most.
What is gradient in multivariable calculus?
The gradient is a fancy word for derivative, or the rate of change of a function. It’s a vector (a direction to move) that. Points in the direction of greatest increase of a function (intuition on why)

What is the gradient of a multivariable function?
In the case of scalar-valued multivariable functions, meaning those with a multidimensional input but a one-dimensional output, the answer is the gradient. The gradient of a function f, denoted as ∇ f \nabla f ∇f , is the collection of all its partial derivatives into a vector.
What does steep ascent mean?
Meaning of word steep ascent in English – English Dictionary. climbing up something with a sharp inclination, inclined ascension which is difficult to perform.
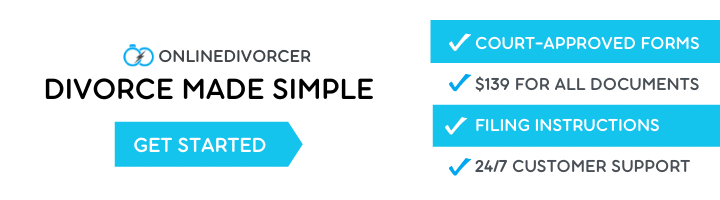
What is stochastic batch or mini batch gradient descent?
Mini Batch Gradient Descent SGD can be used when the dataset is large. Batch Gradient Descent converges directly to minima. SGD converges faster for larger datasets. But, since in SGD we use only one example at a time, we cannot implement the vectorized implementation on it. This can slow down the computations.
What is difference between stochastic batch and mini batch gradient descent?
In the case of Stochastic Gradient Descent, we update the parameters after every single observation and we know that every time the weights are updated it is known as an iteration. In the case of Mini-batch Gradient Descent, we take a subset of data and update the parameters based on every subset.
What are 4 types of slopes?
Slopes come in 4 different types: negative, positive, zero, and undefined. as x increases. The slope of a line can also be interpreted as the “average rate of change”.
What is gradient science?
gradient From the word “grade,” it describes the incline, slope or degree of increase in some measure (such as temperature, pressure or even color) that develops as one moves in time, position or along some scale.
How do you find the gradient of two variables?
For a function of two variables f(x, y), the gradi- ent Vf = is a vector valued function of x and y. At a point (a, b), the gradient is a vector in the xy-plane that points in the direction of the greatest increase for f(x, y). 1.3. Functions of three variables.
What ascent means?
Definition of ascent 1a : the act of rising or mounting upward : climb completed their ascent of the mountain. b : an upward slope or rising grade : acclivity followed the steep ascent to the top of the hill. c : the degree of elevation : inclination, gradient.
What is ascent example?
a : the act or process of rising, moving, or climbing up — usually singular. Fifty years ago, he made the first successful ascent of the mountain. [=he was the first person to climb the mountain] The climbers completed their ascent to the mountain on a rainy morning in April.
What is gradient in data science?
A gradient simply measures the change in all weights with regard to the change in error. You can also think of a gradient as the slope of a function. The higher the gradient, the steeper the slope and the faster a model can learn. But if the slope is zero, the model stops learning.
What is convex function in machine learning?
A function f is said to be a convex function if the seconder-order derivative of that function is greater than or equal to 0. Condition for convex functions. Examples of convex functions: y=eˣ, y=x². Both of these functions are differentiable twice.