What do you do if your residuals are not normally distributed?
Table of Contents
What do you do if your residuals are not normally distributed?
When these don’t show up in your data it’s going to ‘fail’ the normality tests. So rather than relying on the tests, plot the residuals and look to see if they look approximately normal. You will see this method showing up in papers without them using a normality-test that gives an exact p-value.
What do you do if your dependent variable is not normally distributed?
In short, when a dependent variable is not distributed normally, linear regression remains a statistically sound technique in studies of large sample sizes. Figure 2 provides appropriate sample sizes (i.e., >3000) where linear regression techniques still can be used even if normality assumption is violated.
What does non normally distributed residuals mean?
When the residuals are not normally distributed, then the hypothesis that they are a random dataset, takes the value NO. This means that in that case your (regression) model does not explain all trends in the dataset.

Do residuals need to be normally distributed?
Normality of the residuals is an assumption of running a linear model. So, if your residuals are normal, it means that your assumption is valid and model inference (confidence intervals, model predictions) should also be valid.
How do you address non normality?
This review identified at least eight distinct methods suggested to address non-normality, which we organize into a new taxonomy according to whether the approach: (a) remains within the linear model, (b) changes the data, and (c) treats normality as informative or as a nuisance.
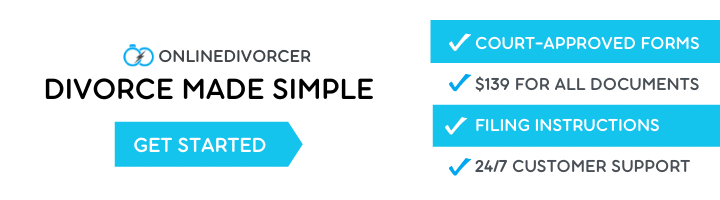
How do you convert non-normal data?
Some common heuristics transformations for non-normal data include:
- square-root for moderate skew: sqrt(x) for positively skewed data,
- log for greater skew: log10(x) for positively skewed data,
- inverse for severe skew: 1/x for positively skewed data.
- Linearity and heteroscedasticity:
Why is normality of residuals important?
The basic assumption of regression model is normality of residual. If your residuals are not not normal then there may be problem with the model fit,stability and reliability. In order to generalize a regression model beyond the sample, it is necessary to check some of the assumptions of regression residuals.
How do I know if my residuals are normally distributed?
You can see if the residuals are reasonably close to normal via a Q-Q plot. A Q-Q plot isn’t hard to generate in Excel. Φ−1(r−3/8n+1/4) is a good approximation for the expected normal order statistics. Plot the residuals against that transformation of their ranks, and it should look roughly like a straight line.
How do you address normality violations?
Data transformation: A common issue that researchers face is a violation of the assumption of normality. Numerous statistics texts recommend data transformations, such as natural log or square root transformations, to address this violation (see Rummel, 1988).
What do you do with non normal errors?
When faced with non-normally in the error distribution, one option is to transform the target space. With the right function f, it may be possible to achieve normality when we replace the original target values y with f(y). Specifics of the problem can sometimes lead to a natural choice for f.
How do you force data into a normal distribution?
Taking the square root and the logarithm of the observation in order to make the distribution normal belongs to a class of transforms called power transforms. The Box-Cox method is a data transform method that is able to perform a range of power transforms, including the log and the square root.
How do you know if normality is violated?
Potential assumption violations include:
- Implicit factors: lack of independence within a sample.
- Outliers: apparent nonnormality by a few data points.
- Patterns in plot of data: detecting nonnormality graphically.
- Special problems with small sample sizes.
- Special problems with very large sample sizes.
How do you check for normality of errors?
OLS diagnostics: Error term normality
- Sort the residuals.
- Calculate the p-value of standardized residuals.
- Construct a vector of empirical probabilities.
- Plot the cumulative probabilities on the vertical axis against the empirical probabilities.
How do you normalize a distribution?
Converting any distribution to Normal distribution:
- Min Max Scaling.
- (X1 — MIN(X1) )/ MAX(X1) — MIN(X1)
- Standard Score.
- (x1 — μ) / σ
- Where μ = mean and σ = standard deviation.
- Divide by Max.
- x1/max(x1)
- We will therefore normalize the prices distribution by using Divide by Max as following :
What is residual normality test?
Normality is the assumption that the underlying residuals are normally distributed, or approximately so. While a residual plot, or normal plot of the residuals can identify non-normality, you can formally test the hypothesis using the Shapiro-Wilk or similar test.