What is cluster sampling in research examples?
Table of Contents
What is cluster sampling in research examples?
An example of single-stage cluster sampling – An NGO wants to create a sample of girls across five neighboring towns to provide education. Using single-stage sampling, the NGO randomly selects towns (clusters) to form a sample and extend help to the girls deprived of education in those towns.
Is cluster sampling a random sampling method?
Cluster sampling divides the population into groups, then takes a random sample from each cluster. Both systematic sampling and cluster sampling are forms of random sampling, known as probability sampling, which stands in contrast to non-probability sampling.
What is cluster simple random sampling?
A cluster random sampling is a probability sampling method where researchers divide the population into clusters or smaller groups. The researcher then randomly selects among the cluster samples to conduct research and collect data.

What type of research uses cluster sampling?
Cluster sampling is typically used in market research. It’s used when a researcher can’t get information about the population as a whole, but they can get information about the clusters. For example, a researcher may be interested in data about city taxes in Florida.
What is an example of a cluster?
The most common cluster used in research is a geographical cluster. For example, a researcher wants to survey academic performance of high school students in Spain. He can divide the entire population (population of Spain) into different clusters (cities).
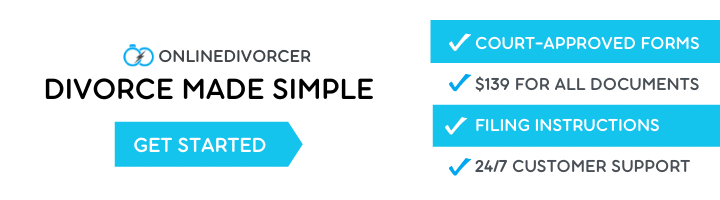
What are the benefits of cluster sampling?
Advantages of Cluster Sampling Since cluster sampling selects only certain groups from the entire population, the method requires fewer resources for the sampling process. Therefore, it is generally cheaper than simple random or stratified sampling as it requires fewer administrative and travel expenses.
What are the three types of cluster sampling?
There are three types of cluster sampling: single-stage, double-stage and multi-stage clustering. In all three types, you first divide the population into clusters, then randomly select clusters for use in your sample.
What are advantages of cluster sampling?
Why is cluster sampling important?
Cluster sampling is a great way for researchers to study an entire population – without having to survey the entire population. It’s cost-effective, efficient, offers easier analysis, and is generally very reliable.
What are the advantages of cluster sampling?
What are the advantages and disadvantages of cluster?
The main advantage of a clustered solution is automatic recovery from failure, that is, recovery without user intervention. Disadvantages of clustering are complexity and inability to recover from database corruption.
What are the strengths and weaknesses of cluster sampling?
List of the Advantages of Cluster Sampling
- It allows for research to be conducted with a reduced economy.
- Cluster sampling reduces variability.
- It is a more feasible approach.
- Cluster sampling can be taken from multiple areas.
- It offers the advantages of random sampling and stratified sampling.
What are the advantages and disadvantages of cluster analysis?
There are various advantages as well, such as it does not require the knowledge of the number of clusters present. And the disadvantage is that it depends on the scale of data. There are two types of approaches used in this kind of Clustering, which are Agglomerative and Divisive.
What are the advantages of cluster random sampling?
What is clustering and its benefits?
Clustering Intelligence Servers provides the following benefits: Increased resource availability: If one Intelligence Server in a cluster fails, the other Intelligence Servers in the cluster can pick up the workload. This prevents the loss of valuable time and information if a server fails.
What is the advantage of cluster random sampling?
What are the advantages of a cluster?
Increased performance: Multiple machines provide greater processing power. Greater scalability: As your user base grows and report complexity increases, your resources can grow. Simplified management: Clustering simplifies the management of large or rapidly growing systems.
What is the difference between stratified and cluster sampling?
In Cluster Sampling, the sampling is done on a population of clusters therefore, cluster/group is considered a sampling unit. In Stratified Sampling, elements within each stratum are sampled. In Cluster Sampling, only selected clusters are sampled. In Stratified Sampling, from each stratum, a random sample is selected.