How does coordinate descent work?
Table of Contents
How does coordinate descent work?
The idea behind coordinate descent methods is simple. If f is a k -dimensional function, we can minimize f by successively minimizing each of the individual dimensions of f in a cyclic fashion, while holding the values of f in the other dimensions fixed.
What is block coordinate descent?
Block coordinate descent (BCD) methods approach optimization problems by performing gradient steps along alternating subgroups of coordinates. This is in contrast to full gradient descent, where a gradient step updates all coordinates simultaneously.
Is coordinate descent the same as gradient descent?
Coordinate descent will update each variable in a Round Robin fashion. Despite the learning rate of the gradient descent procedure (which could indeed speed up convergence), the comparison between the two is fair at least in terms of complexity.

Does coordinate descent converge?
An early application of coordinate descent optimization was in the area of computed tomography where it has been found to have rapid convergence and was subsequently used for clinical multi-slice helical scan CT reconstruction.
What is Admm algorithm?
The alternating direction method of multipliers (ADMM) is an algorithm that attempts to solve a convex optimization problem by breaking it into smaller pieces, each of which will be easier to handle. A key step in ADMM is the splitting of variables, and different splitting schemes lead to different algorithms.
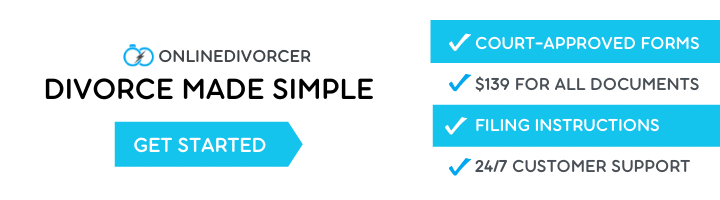
What is stochastic average gradient?
We propose the stochastic average gradient (SAG) method for optimizing the sum of a finite number of smooth convex functions. Like stochastic gradient (SG) methods, the SAG method’s iteration cost is independent of the number of terms in the sum.
What is gradient descent and delta rule?
Gradient descent is a way to find a minimum in a high-dimensional space. You go in direction of the steepest descent. The delta rule is an update rule for single layer perceptrons. It makes use of gradient descent.
Who invented Admm?
The original ADMM algorithm was proposed by Gabay and Mercier [9], and Glowinski, and Marrocco [10]. Many further findings in ADMM were discovered by Eckstein and Bertsekas [11].
What is multiplier method?
Lagrange multiplier method is a technique for finding a maximum or minimum of a function F(x,y,z) subject to a constraint (also called side condition) of the form G(x,y,z) = 0. Figure 1: The four possible cases of varying end points in the direction of y.
Why is Stochastic Gradient Descent better?
According to a senior data scientist, one of the distinct advantages of using Stochastic Gradient Descent is that it does the calculations faster than gradient descent and batch gradient descent. However, gradient descent is the best approach if one wants a speedier result.
Why do we need stochastic approximation to gradient descent?
SGD is much faster but the convergence path of SGD is noisier than that of original gradient descent. This is because in each step it is not calculating the actual gradient but an approximation. So we see a lot of fluctuations in the cost.
What is delta rule used for?
What Does Delta Rule Mean? The Delta rule in machine learning and neural network environments is a specific type of backpropagation that helps to refine connectionist ML/AI networks, making connections between inputs and outputs with layers of artificial neurons. The Delta rule is also known as the Delta learning rule.
What is widrow Hoff rule?
As stated above, the Widrow-Hoff rule aims to minimize the mean square difference between the predicted (expected) and the actual (observed) data or response. In the authors ‘own words “the design objective is the minimization of the average number of errors” (Widrow & Hoff, 1960, p. 96).
What is the difference between AdaGrad and RMSProp?
AdaGrad uses the second moment with no decay to deal with sparse features. RMSProp uses the second moment by with a decay rate to speed up from AdaGrad.
Which Optimizer is best for NLP?
Optimization algorithm Adam (Kingma & Ba, 2015) is one of the most popular and widely used optimization algorithms and often the go-to optimizer for NLP researchers. It is often thought that Adam clearly outperforms vanilla stochastic gradient descent (SGD).