How do you test for heteroskedasticity in SPSS?
Table of Contents
How do you test for heteroskedasticity in SPSS?
TEST STEPS HETEROSKEDASTICITY GRAPHS SCATTERPLOT SPSS
- Activate SPSS program, then click Variable View, then on the Name write X1, X2, and Y.
- Then click Data View, then enter the value for each variable.
- Next step click Analyze – Regression – Linear …
What is Goldfeld Quandt test used for?
The Goldfeld Quandt Test is a test used in regression analysis to test for homoscedasticity. It compares variances of two subgroups; one set of high values and one set of low values. If the variances differ, the test rejects the null hypothesis that the variances of the errors are not constant.
How do you test for heteroscedasticity in regression?

One informal way of detecting heteroskedasticity is by creating a residual plot where you plot the least squares residuals against the explanatory variable or ˆy if it’s a multiple regression. If there is an evident pattern in the plot, then heteroskedasticity is present.
How do you interpret heteroscedasticity?
One of the most common ways of checking for heteroskedasticity is by plotting a graph of the residuals. Visually, if there appears to be a fan or cone shape in the residual plot, it indicates the presence of heteroskedasticity.
How do you fix heteroscedasticity?
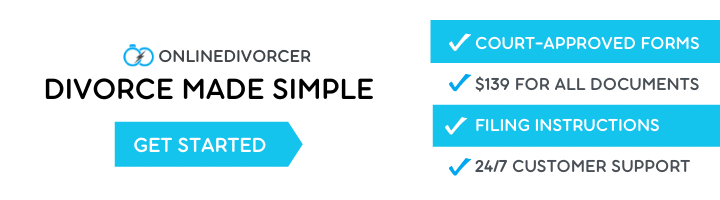
Another way to fix heteroscedasticity is to use weighted regression. This type of regression assigns a weight to each data point based on the variance of its fitted value. What is this? Essentially, this gives small weights to data points that have higher variances, which shrinks their squared residuals.
How do you cure heteroscedasticity?
Remedies for Heteroscedasticity
- Use OLS estimator to estimate the parameters of the model. Correct the estimates of the variances and covariances of the OLS estimates so that they are consistent.
- Use an estimator other than the OLS estimator to estimate the parameters of the model.
Is heteroscedasticity good or bad?
Heteroskedasticity has serious consequences for the OLS estimator. Although the OLS estimator remains unbiased, the estimated SE is wrong. Because of this, confidence intervals and hypotheses tests cannot be relied on. In addition, the OLS estimator is no longer BLUE.
What is homoscedasticity in regression analysis?
Homoskedastic (also spelled “homoscedastic”) refers to a condition in which the variance of the residual, or error term, in a regression model is constant. That is, the error term does not vary much as the value of the predictor variable changes.
Why is homoscedasticity important in regression analysis?
Assumptions. Here are some important assumptions of linear regression. The primary assumption is residuals are homoscedastic. Homoscedasticity means that they are roughly the same throughout, which means your residuals do not suddenly get larger.