What is exploratory factor analysis?
Table of Contents
What is exploratory factor analysis?
Exploratory factor analysis (EFA) is generally used to discover the factor structure of a measure and to examine its internal reliability. EFA is often recommended when researchers have no hypotheses about the nature of the underlying factor structure of their measure.
What is exploratory factor analysis example?
Examples of measured variables could be the physical height, weight, and pulse rate of a human being. Usually, researchers would have a large number of measured variables, which are assumed to be related to a smaller number of “unobserved” factors.
How do you conduct an exploratory factor analysis?
First go to Analyze – Dimension Reduction – Factor. Move all the observed variables over the Variables: box to be analyze. Under Extraction – Method, pick Principal components and make sure to Analyze the Correlation matrix. We also request the Unrotated factor solution and the Scree plot.

What is a CFA model?
CFA allows for the assessment of fit between observed data and an a prioriconceptualized, theoretically grounded model that specifies the hypothesized causal relations between latent factors and their observed indicator variables.
What is exploratory factor analysis in SPSS?
Exploratory factor analysis is a statistical technique that is used to reduce data to a smaller set of summary variables and to explore the underlying theoretical structure of the phenomena. It is used to identify the structure of the relationship between the variable and the respondent.
What is difference between EFA and CFA?
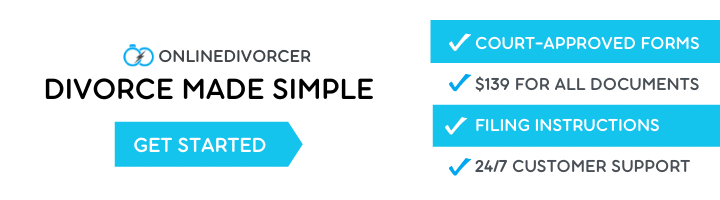
General rule: EFA > Used for instruments (or scales) that have never been tested before (for their validity are reliability). CFA > Used for instruments (or scales) that have been tested before (for their validity are reliability).
Should I use factor analysis or PCA?
If you assume or wish to test a theoretical model of latent factors causing observed variables, then use factor analysis. If you want to simply reduce your correlated observed variables to a smaller set of important independent composite variables, then use PCA.
What is EFA CFA and SEM?
Using EFA (or similar process) you come to derive the constructs for the groups of these items. CFA is used to confirm and trim these constructs and items (measurement model). SEM is used to find if relationships exist between these items and constructs (structural model).
What is the difference between PCA and EFA?
PCA and EFA have different goals: PCA is a technique for reducing the dimensionality of one’s data, whereas EFA is a technique for identifying and measuring variables that cannot be measured directly (i.e., latent variables or factors).
Why is Bartlett test used?
Bartlett’s test (Snedecor and Cochran, 1983) is used to test if k samples have equal variances. Equal variances across samples is called homogeneity of variances. Some statistical tests, for example the analysis of variance, assume that variances are equal across groups or samples.
What does the Bartlett test show?
Bartlett’s test is used to test the null hypothesis, H0 that all k population variances are equal against the alternative that at least two are different. is the pooled estimate for the variance.
What is the difference between confirmatory and exploratory factor analysis?
In exploratory factor analysis, all measured variables are related to every latent variable. But in confirmatory factor analysis (CFA), researchers can specify the number of factors required in the data and which measured variable is related to which latent variable.