What is the difference between frequentist and Bayesian approaches?
Table of Contents
What is the difference between frequentist and Bayesian approaches?
Frequentist statistics never uses or calculates the probability of the hypothesis, while Bayesian uses probabilities of data and probabilities of both hypothesis. Frequentist methods do not demand construction of a prior and depend on the probabilities of observed and unobserved data.
What is the difference between Bayesian and frequentist approach for machine learning?
Practically, in machine learning a model is a formula with tunable parameters. Then the difference between Bayesian and frequentist is: That the parameters are assumed to be fixed numbers in frequentist setting and the parameters have their own distributions in the Bayesian setting.
What are the two approaches to statistics?
Two main statistical methods are used in data analysis: descriptive statistics, which summarize data from a sample using indexes such as the mean or standard deviation, and inferential statistics, which draw conclusions from data that are subject to random variation (e.g., observational errors, sampling variation).

Why is Bayesian statistics better than frequentist?
: the frequentist approach assigns probabilities to data, not to hypotheses, whereas the Bayesian approach assigns probabilities to hypotheses. Furthermore, Bayesian models incorporate prior knowledge into the analysis, updating hypotheses probabilities as more data become available.
What makes Bayesian statistics different from other kinds of statistics?
In contrast Bayesian statistics looks quite different, and this is because it is fundamentally all about modifying conditional probabilities – it uses prior distributions for unknown quantities which it then updates to posterior distributions using the laws of probability.
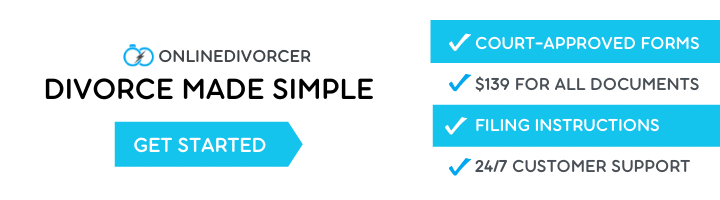
What is Bayesian statistics used for?
Bayesian statistics is a particular approach to applying probability to statistical problems. It provides us with mathematical tools to update our beliefs about random events in light of seeing new data or evidence about those events.
What is Bayesian statistics?
Bayesian statistics is a system for describing epistemological uncertainty using the mathematical language of probability. In the ‘Bayesian paradigm,’ degrees of belief in states of nature are specified; these are non-negative, and the total belief in all states of nature is fixed to be one.
What is Frequentist approach of probability?
The frequentist school of thought holds that probability can only express something about the real world in the context of a repeatable experiment. The frequency of a particular observation converges as more observations are gathered; this limiting value is then called the probability.
What is one of the drawbacks of frequentist statistics?
However, the frequentist method also has certain disadvantages: The required traffic volume does not allow tests to be run in all circumstances. Obtaining statistically significant results when we run A/B tests on pages with low traffic can be difficult or take a long time.
What are the advantages of Bayesian statistics?
Some advantages to using Bayesian analysis include the following: It provides a natural and principled way of combining prior information with data, within a solid decision theoretical framework. You can incorporate past information about a parameter and form a prior distribution for future analysis.
What is the purpose of Bayesian statistics?
What is a frequentist model?
Frequentist Methodology In a frequentist model, probability is the limit of the relative frequency of an event after many trials. In other words, this method calculates the probability that the experiment would have the same outcomes if you were to replicate the same conditions again.
What is likelihood in Bayes Theorem?
Likelihood refers to the probability of observing the data that has been observed assuming that the data came from a specific scenario.
What is meant by Bayesian approach?
The Bayesian approach described is a useful formalism for capturing the assumptions and information gleaned from the continuous representation of the sample values, the histograms calculated from them, and the partial-volume effects of imaging.
What is Bayesian methods for data analysis?
Bayesian analysis, a method of statistical inference (named for English mathematician Thomas Bayes) that allows one to combine prior information about a population parameter with evidence from information contained in a sample to guide the statistical inference process.
Where is Bayesian statistics used?
Simply put, in any application area where you have lots of heterogeneous or noisy data or anywhere you need a clear understanding of your uncertainty are areas that you can use Bayesian Statistics.
What is frequentist theory?
Frequentist probability or frequentism is an interpretation of probability; it defines an event’s probability as the limit of its relative frequency in many trials (the long-run probability). Probabilities can be found (in principle) by a repeatable objective process (and are thus ideally devoid of opinion).
What is frequentist analysis?
Frequentism is the study of probability with the assumption that results occur with a given frequency over some period of time or with repeated sampling. As such, frequentist analysis must be formulated with consideration to the assumptions of the problem frequentism attempts to analyze.
What are the limitations of Bayesian statistics?
There are also disadvantages to using Bayesian analysis: It does not tell you how to select a prior. There is no correct way to choose a prior. Bayesian inferences require skills to translate subjective prior beliefs into a mathematically formulated prior.
Why is the likelihood principle important?
The importance of the likelihood principle is that it discusses if the comparison is not relevant. LP does rule out many specific inferences.