What is parallelepiped classification?
Table of Contents
What is parallelepiped classification?
Parallelepiped classification uses a simple decision rule to classify hyperspectral data. The decision boundaries form an n-dimensional parallelepiped in an image data space. The dimensions of a parallelepiped classifier are defined based on the standard deviation threshold from the mean of each selected class.
What is image classification in remote sensing?
In a broad sense, image classification is defined as the process of categorizing all pixels in an image or raw remotely sensed satellite data to obtain a given set of labels or land cover themes (Lillesand, Keifer 1994).
What is image give the classification of image?
Image classification is the process of categorizing and labeling groups of pixels or vectors within an image based on specific rules. The categorization law can be devised using one or more spectral or textural characteristics. Two general methods of classification are ‘supervised’ and ‘unsupervised’.
Why image classification is important in remote sensing?

Image Classification techniques are used to categorize the pixels in the satellite data for differentiating the various earth features such as barren land, forests, roads, settlements, water bodies, rocks based on the spectral reflectance.
What is parallelepiped in remote sensing?
The parallelepiped classifier is one of the widely used supervised classification algorithms for multispectral images. The threshold of each spectral (class) signature is defined in the training data, which is to determine whether a given pixel within the class or not.
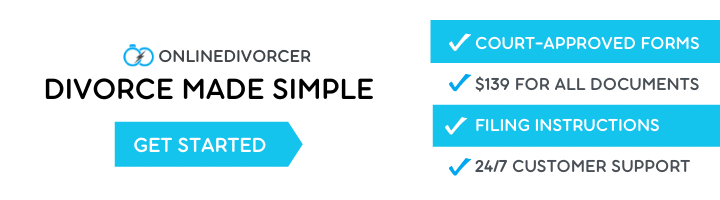
What is a classifier in image processing?
Image classification refers to the task of extracting information classes from a multiband raster image. The resulting raster from image classification can be used to create thematic maps.
What are the classification of remote sensing?
There exist two main types of remote sensing classified according to the source of signal they use to explore the object, active vs. passive. Active remote sensing instruments operate with their own source of emission or light, while passive ones rely on the reflected one.
What is image classification GIS?
Image classification refers to the task of assigning classes—defined in a land cover and land use classification system, known as the schema—to all the pixels in a remotely sensed image. The output raster from image classification can be used to create thematic maps.
Which is better for image classification?
It depends on your object of classification. If color has no significance in your images to classify then its better to go for grey scale images to avoid false classification and complexities.
Why is image classification used?
The objective of image classification is to identify and portray, as a unique gray level (or color), the features occurring in an image in terms of the object or type of land cover these features actually represent on the ground. Image classification is perhaps the most important part of digital image analysis.
Which classifier is best for image classification?
Convolutional Neural Networks (CNNs) is the most popular neural network model being used for image classification problem.
What is the purpose of a classifier?
In data science, a classifier is a type of machine learning algorithm used to assign a class label to a data input. An example is an image recognition classifier to label an image (e.g., “car,” “truck,” or “person”).
What is supervised and unsupervised image classification?
The image pixel has been employed as the basic unit of analysis since the early 1980s. The supervised and unsupervised image classification techniques are considered the major categories. Supervised is mainly a human-guided classification. In contrast, unsupervised classification is calculated by the software.
Is image classification supervised or unsupervised?
Image classification is mainly divided into two categories (1) supervised image classification and (2) unsupervised image classification. In supervised image classification training stage is required, which means first we need to select some pixels form each class called training pixels.
How many layers are there in image classification?
There are three types of layers in Convolutional Neural Networks: 1) Convolutional Layer: In a typical neural network each input neuron is connected to the next hidden layer.